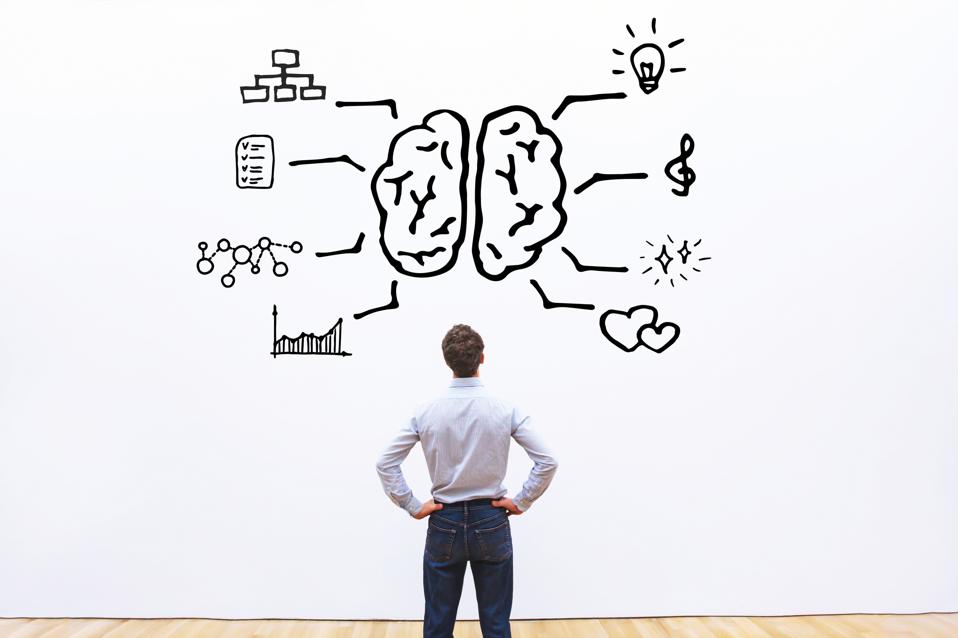
Figuring out how the human brain works.
Your brain and mind make you who you are. And how they’re built is unique to you. That’s not a figure of speech, it’s the literal truth. The precise wiring of your brain - how your billions of neurons are connected - is different from everyone else.
Yet, despite this, interacting with other people and their own distinctive brains is an everyday occurrence. Understanding how the brain has evolved to communicate with other different brains - identifying universal computational rules and algorithms despite the diversity in how they’re wired up - has proven difficult to solve. Mathematics is providing the tools necessary to do so. In the process, it also has the potential to advance machine learning and artificial intelligence.
The genes in your cells encode a blueprint that resulted in your brain having roughly the same function and organization as most other people. Different brain regions do similar things and are connected up in about the same way. But the genetic blueprint can’t specify the precise connection patterns between all the individual neurons in your brain. It can’t encode enough information to precisely and deterministically dictate every single connection (synapses) of the roughly 10 quadrillion that make up the massive network of neurons that is the brain.
Beyond the information that is encoded in your genes, the final connectivity patterns between neurons make use of random (stochastic) processes that are influenced by environmental conditions and stressors - how you interact with the external world and how the world interacts with you. As a result, how your brain takes in and processes information is also unique to who you are as an individual. Yet, the brains of different people are able to support roughly the same kinds and degrees of computations and functions. The question is how?
Why Mathematics Is Necessary to Understand How the Brain Works
The brain is incredibly complex. It is beyond complicated. It is truly complex in the sense that the whole is greater than the sum of the parts. Even though scientists know quite a lot about the biology, physics, and chemistry that make up the cells in the brain and how they function and interact with each other, they still have comparatively little understanding about how the global functions of the brain emerge. Things like cognition, imagination, emotions, and even consciousness.
Given the degree of complexity involved, scientists need to use mathematics as a unifying language and framework to keep track of all the details that are known about the brain, and how those details interact with each other.
Experiments generate data and details about how the brain works across many scales of organization - genetic, molecular, cellular, networks, all the way up to cognitive and behavioral. They are the workhorses necessary to understand the myriad of processes that underlie how the brain functions. After all, neuroscience, like all the natural sciences, is an experimental pursuit.
However, it is difficult to arrive at any meaningful understanding of the brain computations that give rise to emergent cognitive properties by considering individual experimental details in isolation.
Mathematical methods provide a toolset capable of keeping track of all the details and, importantly, how dynamic interactions among them influence one another in the face of contextually changing situations your brain is exposed to. There are simply too many moving parts, and too many details to keep track of, such that it would be impossible to do so without the power that mathematics brings to bear.
What’s more, putting neurobiological details into a mathematical framework provides not only a description of the brain, but also supports making predictions. Ideally, such predictions will be experimentally testable so they can be verified - or disproven - by experiments. One of the most striking successes of this approach was Hodgkin and Huxley’s Nobel Prize winning work to understand the action potential - the basis for how neurons communicate with each other via discrete electrical impulses.
To be clear though, the data provided by experiments is critical for informing mathematical models. The descriptive and predictive capabilities of mathematics applied to any physical system, including the brain, will only ever be as good as the experimental data that informs it in the first place. So high quality experiments are critical.
It is also important to appreciate that mathematical models of the brain do not need to reverse engineer every detail it uses to carry out its computations.
The ‘stuff’ the brain is made from, its ‘wetware’, necessarily dictates and constrains how biology is able to carry out those computations. But the rules themselves - the brain’s algorithms - are independent of the wetware. From a mathematical perspective, a distinction can be made between a mathematical description that captures brain algorithms, for example as set of equations, versus the wet and squishy biology and chemistry that the brain needs to use because it has to.
In essence, such mathematical descriptions abstract away unnecessary details about the biological implementation. If you can describe brain computations and algorithms purely mathematically, then it also opens up the opportunity for an understanding of the brain to inform machine learning and artificial intelligence.
And by extension, the use of mathematics to understand how the brain works also provides an opportunity to understand changes associated with complex neurological, neurodevelopmental, and psychiatric disorders. For example, Alzheimer’s disease and other dementias, epilepsy, and autism spectrum disorder. While clinically and pathophysiologically distinct, they all share features associated with genetic and molecular changes that ultimately affect network function and information processing.
Uncovering the Mathematical Rules of Brain Function
A number of research groups around the world, using various mathematical techniques, are engaged in an attempt to understand how universal functional invariance in the brain - in other words, a common capacity - occurs given the large degree of random variability displayed by the structural connectivity of neurobiological networks.
Addressing this is no small task, and a number of key decisions about how the problem is approached need to be made before scientists can attempt to make progress. For example, what are the right mathematical tools to use? How much of the biology should be ignored - abstracted away - and how much of it should one keep in order to capture and preserve the key ideas and principles that explain such functional invariance?
And once you do develop an appropriate mathematical model, how generalizable is the resultant theory, say, to explaining neurological disorders, or towards applying it to machine learning and artificial intelligence?
For example, a pre-print paper posted just a few days ago (meaning it’s still a work in progress and has not yet been published in a peer review journal) looked at genetically encoded rules that specify how neurons of different classes statistically decide to connect with each other.
The authors examined published experimental data and took note of key measurements and relationships associated with how neurons make such connection decisions. They then ‘translated’ those observations into a set of mathematical rules - similar to how a recipe specifies how a set of ingredients need to come together and in what proportions in order to make a cake. The ‘cake’ in this case was a neural circuit capable of performing certain kinds of basic computations. The ‘ingredients’ were the number of different classes of neurons, the chances (probability) that two neurons of different classes connect with each other, how prevalent those classes are, and the ‘strength’ or weight of the connection between them. The notion of a weight here conceptually captures the amount of influence one neuron has on another.
Using their mathematical model, the authors were able to show in example test cases that the set of genetically encoded rules they took into account were sufficient to allow neural circuits to carry out meaningful, albeit basic, computational tasks.
In contrast, other work has focused not on the genetic code, but rather how spontaneous neural activity - billions of electrical impulses - shape how networks and circuits of interconnected neurons ultimately wire up. Different patterns of activity can influence the stable connectivity patterns of mature neural circuits.
Such activity-dependent priming for how neurons connect with each other is critical actually. It’s known that if the right kind of activity at the right time during development is absent it can affect the brain’s normal development. In turn, this can result in improper brain function later on in life.
There’s no question that there’s a long road ahead for scientists to fully understand how the brain works - if they ever get there at all. But what’s certain is that it will require the use of mathematics to do so. After all, despite its mysterious and awe inspiring nature the brain is still a physical structure subject to physical rules. The Universe may speak every language with equal fluidity, but its native tongue is mathematics.