Partner at DeciBio Consulting, fostering a community to accelerate the commercialization of disruptive personalized medicine technologies.
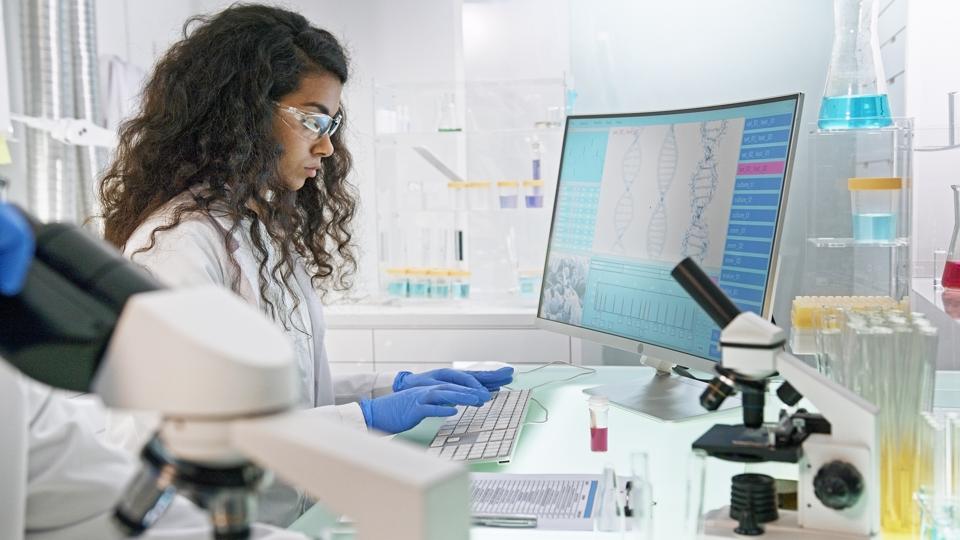
getty
I believe precision medicine has the potential to drastically improve health care outcomes. Nevertheless, without careful consideration for improving health care across demographics, new technologies backed by artificial intelligence may exacerbate systemic racial disparities in health care. Algorithms primed to detect a signal that “works” for a majority of people may underperform for historically underrepresented groups and contribute to or sustain current disparities in the health care system. Even though low socioeconomic status (SES) groups have invested in improving health care at large through participation in clinal trials, research suggests that health care outcomes are worse for lower-SES groups. I believe these systemic inequities contribute to current distrust in the health care system and could be exacerbated by AI algorithms.
Historically, throughout the process of scientific advancement, lower-SES and minority groups have borne some of the greatest risks. Beginning in 1932, the infamous Tuskegee study followed a cohort of untreated Black men with syphilis. Additionally, we continue to derive benefit from the contributions of Henrietta Lacks, a Black woman whose cancerous germline cells (“HeLa” cells) were distributed without her permission. Today, people of color may be more likely to be serial participants in the most risky phases of clinical trials, such as phase one trials, that may have no medical benefit for the participant but rather focus on evaluating the safety of a new drug candidate on healthy volunteers. For example, a recent survey by Jill Fischer (via Harvard University) found that, of clinical trial participants surveyed, "all of the volunteers who had participated in more than 50 trials (in total six) were Black men and more than a third of the Black volunteers she interviewed had participated in more than 10 trials." She estimated that Black people made up 35% of phase one participants, yet they make up about 13% of the U.S. population. Despite this, the American Medical Association reports that the racial divide in health care outcomes persists, which may contribute to distrust in the U.S. health care system. In fact, 2020 research from The Undefeated and The Kaiser Family Foundation covered in National Geographic (paywall) found that 55% of Black Americans distrust the health care system. Those working on advancements in precision medicine should create intentional strategies to avoid contributing to the polarization of health care outcomes.
AI algorithms have the potential to unintentionally contribute to racial inequities in health care outcomes when there are significant genetic differences like epigenetic markers and sequence changes between ethnic groups. For example, triple-negative breast cancer (TNBC) is more prevalent among non-white populations, and even subtypes of TNBC have racially biased incidence rates. Nevertheless, as explained in Cancer Discovery, AI algorithms may control for any “stray” signal by including race as a variable in the regression model. By blunting the noise in the dataset when an insignificant number of minority groups are represented, the algorithm may fail to detect disease, thus catering a health care tool to a majority-white population. Google CEO Sundar Pichai highlighted the importance of understanding backbone models of all algorithms to ensure that they do not emphasize existing biases. If AI algorithms are increasingly integrated into routine health care, such as in cancer screenings, they could exacerbate existing inequities in quality of care.
The increasing implementation of AI in research merits an increase in attention from companies and organizations to how these algorithms can contribute to race-based health care disparities. For example, wearable devices have important implications for data collection and health care monitoring, but research suggests that some may perform better on lighter skin colors. According to the World Health Organization, detecting cancer earlier improves patient prognosis, but as The Atlantic explains, AI-based early detection assays for cancers like melanoma, for example, may perform worse for people of color. Furthermore, in oncology, omitting stray signals in algorithms may minimize research investment into those specific mutations or genomic signatures and lead to a decrease in the development of targeted therapies for that mutation. Neglecting to account for variances between racial groups in oncology, as well as other realms of health care, may result in further health care disparities.
Organizations and companies that incorporate a diverse patient population in their cohorts may be ultimately more successful in reaching a broader patient base and, consequentially, customer base. Groups like the Texas Center for Health Disparities have implemented pilot studies to support data collection and research for the development of therapies for diseases affecting minority groups like TNBC in Black women. Additionally, by identifying optimal subpopulations for therapies, pharmaceutical companies could help ensure therapeutic success and increase revenue overall. For example, tyrosine kinase inhibitors (TKIs) have shown better efficacy in treating those of East Asian descent with non-small cell lung cancer compared to other population cohorts. Likewise, Zytiga, a hormone treatment for metastatic castration-resistant prostate cancer, proved more successful in one study for the treatment of Black men than Caucasian men. By exploring treatment in these diverse populations, companies may be able to prove the efficacy of a therapy that may have demonstrated limited success if only observed in a majority-group-based cohort. I believe extensive research into the race-based nuances of disease types and associated therapies could ultimately increase the treatment-specific addressable market.
Access to health care for all people is critical. However, this access will be more influential if the health care provided adequately addresses all populations it serves. Recent advancements in precision medicine ranging from targeted cancer therapies to the development of AI/ML algorithms for disease detection are not immune to systemic disparities in medicine. If left unguided, these advancements could exacerbate the polarization of health care outcomes by limiting the availability or success of key targeted therapies. Moreover, companies could fall short of their full potential. However, by recognizing and actively correcting for potential new biases that arise, the industry can ensure that precision medicine is truly personalized to all, rather than a select few.
Forbes Business Council is the foremost growth and networking organization for business owners and leaders. Do I qualify?